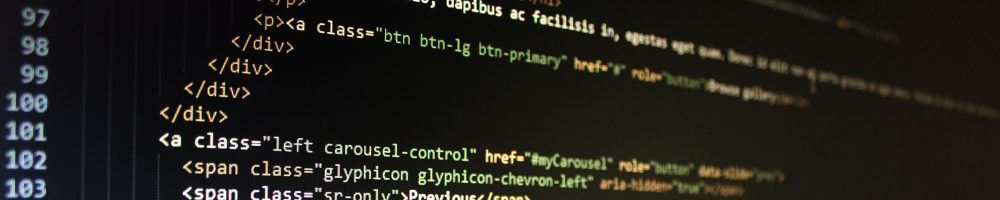
Resources
Brain-state extraction algorithm based on the state transition (BEST)
A fully automatic dynamic functional brain network analysis in fMRI study
Features
- Constructs connectivity matrices from the duration of brain-states and decodes the proper number of brain-states in a data-driven way
- To set the duration of each brain-state, BEST detects brain-state transition time-points using spatial standard deviation of the brain activity pattern that changes over time
- Using Bayesian information criterion (BIC) to the clustering method to estimate and extract the number of brain-states
Download
References
Lee, Y-B., Yoo K., Roh, J.H., Moon, W.J., Jeong, Y. (2019), Brain-state extraction algorithm based on the state transition (BEST): a dynamic functional brain network analysis in fMRI study. Brain Topography 32 (5), 897-913
Contact
For technical questions and feedback, please send an email to Dr. Lee yblee85(at)ibs.re.kr
Data Sharing
Exploring Feature Dimensions to Learn a New Policy in an Uninformed Reinforcement Learning Task
Features
- Exploration of multi-dimensional features in an uninformed reinforcement-learning task.
- Two sets of behavioral experiments (SET1. MRI taken, SET2. behavior only) and fMRI GLM results are included.
- Exploration and transfer learning behavior were examined from SET1 (n=29) and validated from SET2 (n=29).
- Conducted GLM analysis with three model-based parameters (Value, Error, and Cognitive Entropy).
- Single threshold (Small volume correction (SVC) peak-level p<0.05 within combined ROI masks) is used.
Download
- Exploration_ScientificReport_DataSharing.zip
- Include behavioral results, GLM results, and ROI masks
References
Choung, O. H., Lee, S. W., and Jeong, Y. (2017), Exploring Feature Dimensions to Learn a New Policy in an Uninformed Reinforcement Learning Task. Sci. Rep., 7(1): 17676. doi:10.1038/s41598-017-17687-2
Contact
For technical questions and feedback, please send an email to Oh-hyeon Choung - iohyeonki(at)gmail.com
Degree-based Statistics
Degree-based statistic for group network comparison and center persistency for brain connectivity analysis
Features
- Perform a cluster-wise inference
- Provide a corrected p-value in testing hypothesis of 2 sample t-test or Pearson's correlation analysis
- New definition of a cluster which helps overcoming two innate drawbacks of cluster-wise correction
- Robust parameter settings for spatial specificity & the arbitrariness of an initial cluster forming threshold
- Provide an easily interpretable and statistically reliable result
Download
- github: https://github.com/rayksyoo/DBS
- Requirement: MATLAB
References
Yoo, K., Lee, P., Chung, M. K., Sohn, W. S., Chung, S. J., Na, D. L., Ju, D. and Jeong, Y. (2017),
Degree-based statistic and center persistency for brain connectivity analysis. Hum. Brain Mapp., 38: 165–181. doi:10.1002/hbm.23352
Contact
For technical questions and feedback, please send an email to Dr. Yoo raybeam(at)kaist.ac.kr
Sparse SPM (SSPM)
Group Sparse-dictionary learning in SPM framework for resting-state functional connectivity MRI analysis
Features
- Coming soon
Download
- Requirement: MATLAB
References
Lee Y., Lee J., Tak S., Lee K., Na DL., Seo S., Jeong Y., Ye J., The Alzheimer's Disease Neuroimaging Initiative (2016),
Sparse SPM: Group Sparse-dictionay learning in SPM framework for resting-state functional connectivity MRI analysis. Neuroimage 125: 1032-1045. doi: 10.1016/j.neuroimage.2015.10.081
Contact
For technical questions and feedback, please send an email to Dr. Lee yblee85(at)kaist.ac.kr